After 15 years in SaaS, I have watched the shift from rule-based systems to more intelligent, autonomous architectures.
While generative AI has made waves for its ability to create content, agentic AI is quietly transforming how SaaS businesses operate. This shift is not loud, but it is deep and foundational.
Agentic AI can make decisions, take action, and adapt. That gives it the power to become a digital operator within your business rather than just another software tool.
What Makes Agentic AI Different for SaaS
Before we explore specific use cases, it's important to understand how agentic AI stands apart from more traditional AI implementations:
- Autonomous action: These systems take action directly within defined parameters without needing human prompts at every step.
- Workflow orchestration: Agents coordinate across teams, tools, and steps, linking together fragmented processes.
- Contextual awareness: They understand broader conditions, not just single inputs.
- Learning and refinement: They get smarter over time, improving outcomes based on feedback and results.

Now let’s walk through five business functions where agentic AI is already delivering real, measurable value.
5 Practical Agentic AI Use Cases in Business Operations
1. Sales: Let AI Handle the Chase While You Close
Most sales teams spend more time managing their pipeline than selling.
Between researching prospects, scoring leads, enriching records, updating CRMs, and following up, selling often comes last.
Agentic AI changes that. It tracks buyer intent, qualifies leads in real time, enriches data from public and private sources, and crafts personalized outreach.
When a lead goes quiet, it checks for context and either revives the conversation or shelves the lead until a better moment.
Zoomed-In Use Case:
A RevOps leader from a Series B startup visits your pricing page twice within 24 hours.
The AI agent identifies this, checks their engagement history, pulls in company insights using Clearbit, and drafts a follow-up email referencing a past webinar.
If the lead replies, the agent logs the activity in your CRM and books a call with a rep.
The call notes are already prepared, including deal history and talking points.
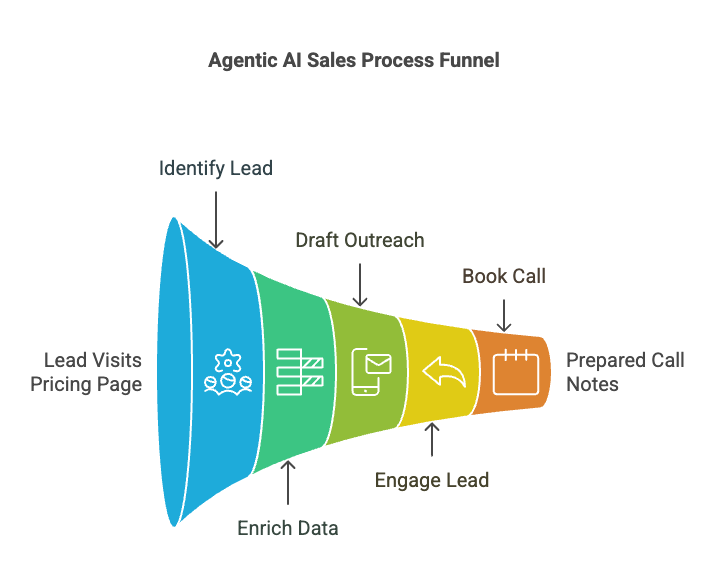
2. Marketing: Newsletters That Write and Send Themselves
Marketing teams spend a lot of time compiling content, segmenting lists, optimizing headlines, and choosing send times.
Agentic AI takes this from routine to real-time. It builds, adapts, personalizes, and ships newsletters based on what users are doing right now.
Zoomed-In Use Case:
The AI scans engagement trends and sees that your mid-market audience is interacting heavily with content on access permissions.
It prepares a newsletter for that segment highlighting a new feature release, adds a tutorial video, and includes a call-to-action for a team-wide training.
For enterprise users, it sends a different version focused on audit trails and compliance.
Each version is sent at the time each segment tends to engage the most.
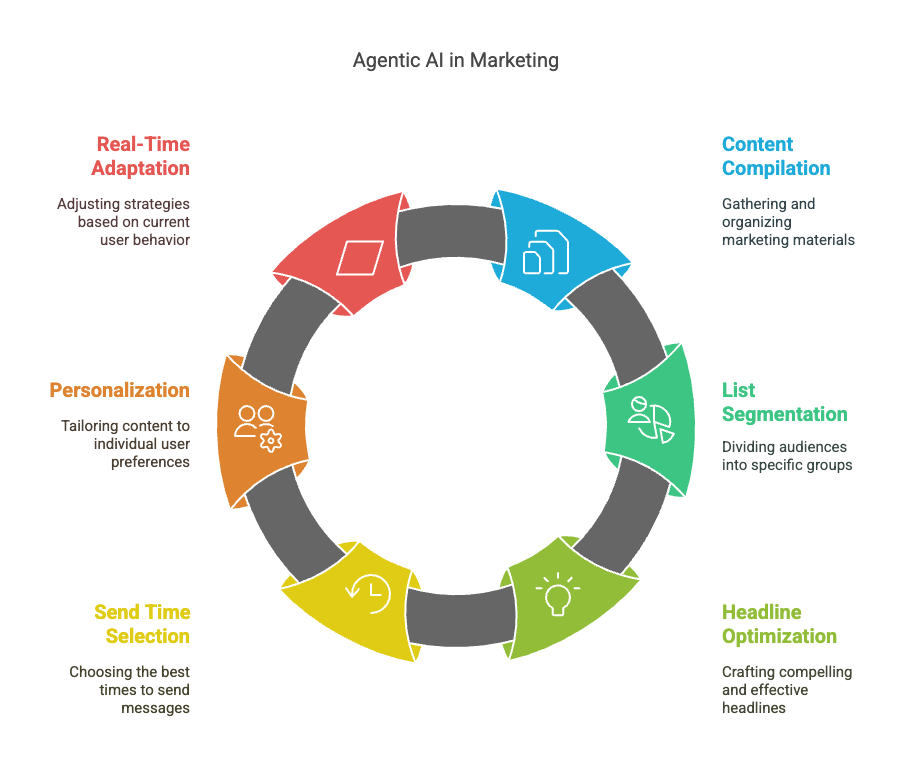
3. Support: Solving Tickets Before They Escalate
Support teams are not buried by complexity but by volume.
Most tickets are repetitive and involve hunting for the same information in different tools. Agentic AI steps in as a first responder.
It checks usage history, reads past tickets, scans logs, and even consults internal documentation before offering a response.
Zoomed-In Use Case:
A user reports that their CRM integration stopped working overnight.
The AI agent checks logs, identifies an expired token, refreshes it, verifies the connection, and confirms the fix in a message to the user.
If the language in the ticket signals growing frustration, the agent tags support leadership.
It also flags the issue to product if similar errors have occurred across accounts.
The entire workflow happens in less than a minute, and no support rep needs to be involved unless escalation is necessary.
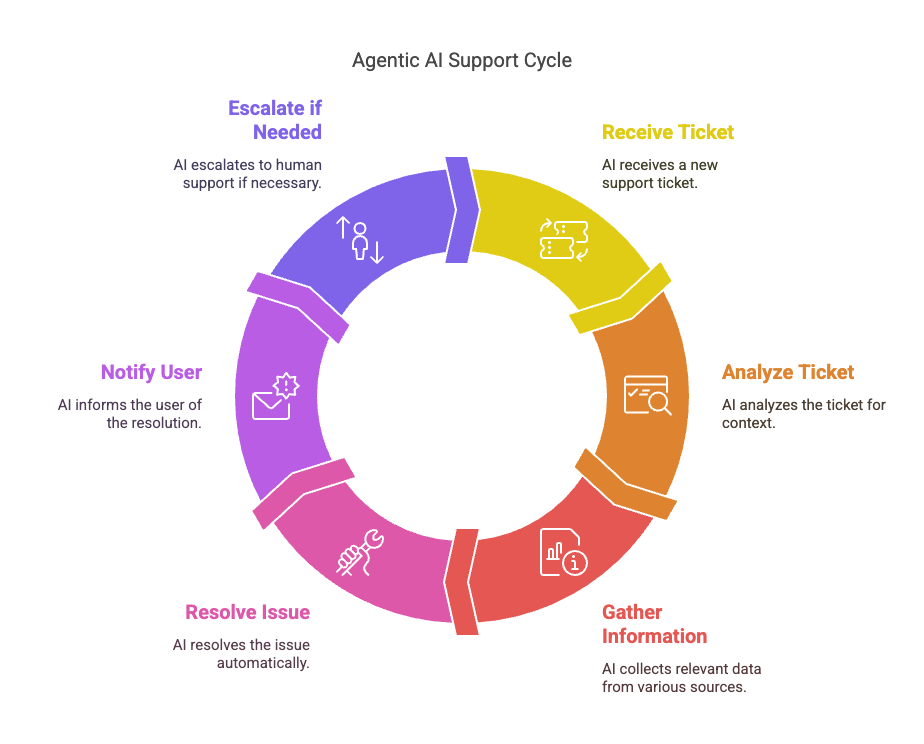
4. Finance: Self-Updating Intelligence
Finance workflows rely on accuracy, timing, and context.
But in many SaaS companies, the systems do not speak to each other, and forecasting is based on outdated information.
Agentic AI changes this. It monitors spend, flags inconsistencies, halts out-of-policy actions, and keeps your forecast current without waiting for a month-end review.
Zoomed-In Use Case:
A sudden increase in contractor spend triggers the AI to investigate.
It finds a new invoice line item that does not match any purchase order.
The agent pauses the transaction, alerts the procurement team in Slack, and proposes a correction.
It also adjusts the financial forecast to reflect the change and attaches documentation for audit readiness.

5. Revenue Operations: One Brain Coordinating the Entire Funnel
RevOps sits at the center of marketing, sales, customer success, and finance.
Its challenge is not in data collection but in connecting the dots and making decisions quickly.
Agentic AI acts like an operations layer that watches everything and acts when needed.
Zoomed-In Use Case:
A lead signs up after attending a product webinar.
The AI notices that the lead was not assigned to a rep due to a territory mismatch.
It corrects the assignment, enriches the record with ZoomInfo data, adds full engagement history to the CRM, notifies the correct rep in Slack, and creates a pipeline task with context from both marketing and product usage.
The lead never falls through the cracks.

Implementation Strategy: Starting Your Agentic AI Journey
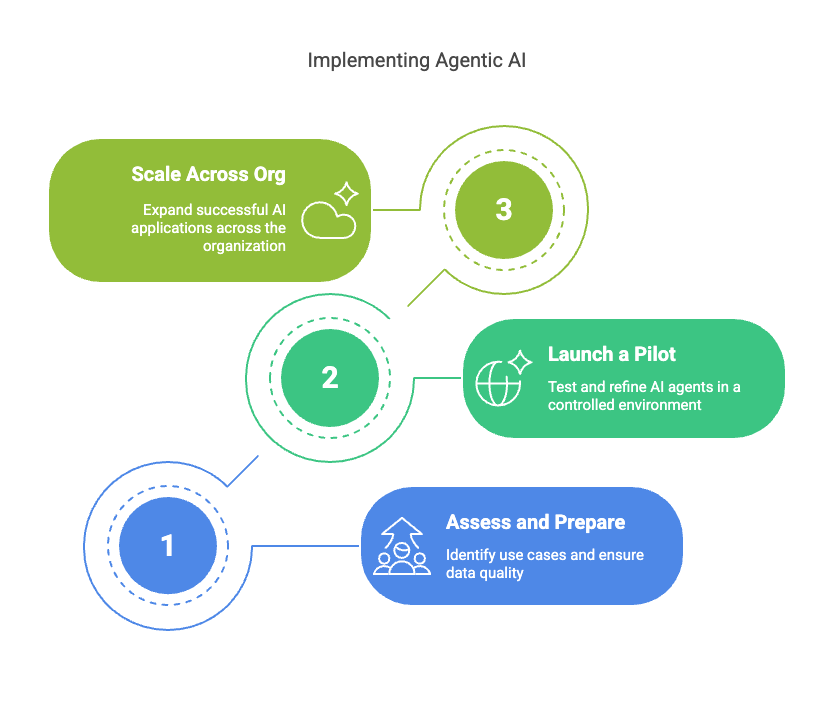
Phase 1: Assess and Prepare
- Identify use cases where agentic AI can drive immediate value
- Check your data quality and how accessible it is across systems
- Choose your technical approach: build in-house, buy off-the-shelf, or work with a partner
- Set up governance around access, action limits, and escalation paths
Phase 2: Launch a Pilot
- Begin with a use case that has clear metrics and impact
- Run the agent in parallel with your existing process to measure the difference
- Use the pilot to tune behavior and build confidence
- Document outcomes, challenges, and lessons learned
Phase 3: Scale Across the Org
- Expand successful use cases into more teams
- Connect your agents across departments to form intelligent workflows
- Train internal teams to manage, monitor, and evolve the agents over time
- Set up regular reviews and feedback loops
Common Challenges and What to Do About Them
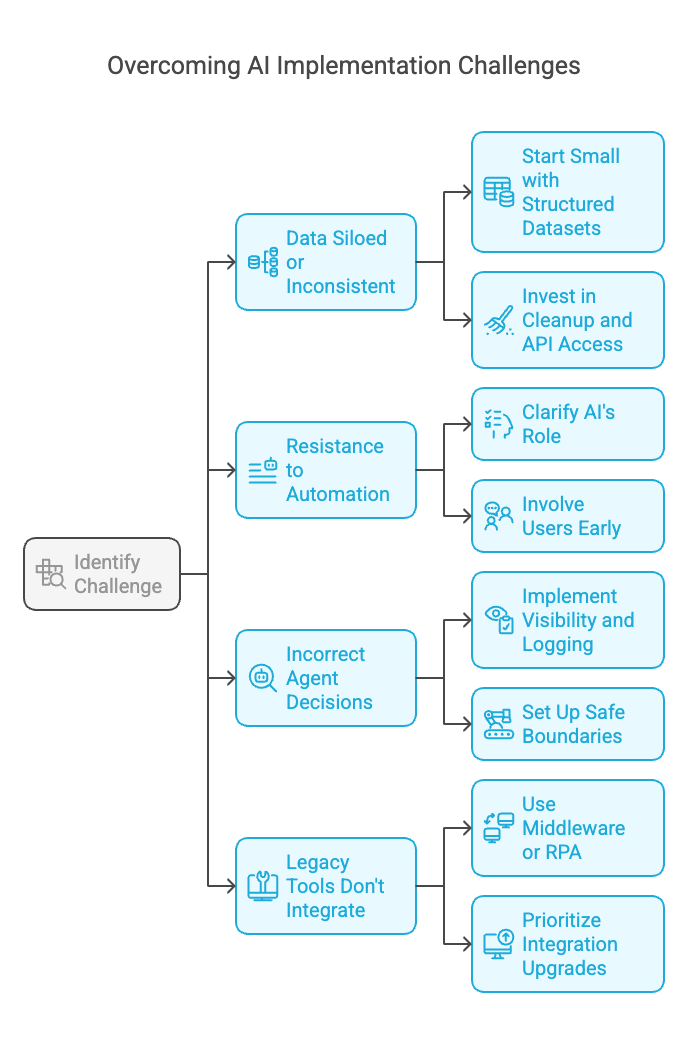
Challenge: Data is siloed or inconsistent
Solution: Start small with well-structured datasets. Invest in cleanup and API access.
Challenge: Resistance to automation
Solution: Make it clear the AI handles routine tasks so teams can focus on impact. Involve users early.
Challenge: Agents make incorrect decisions
Solution: Implement clear visibility, logging, and override options. Set up safe boundaries and learning loops.
Challenge: Legacy tools don’t integrate
Solution: Use middleware or RPA as a bridge. Prioritize integration upgrades based on use case value.
Will Agentic AI Replace SaaS?
It is a valid question, but history tells us that technology waves tend to reshape ecosystems instead of wiping them out.
Mainframes did not disappear when client-server computing arrived.
On-prem systems still exist even after cloud adoption and PCs are alive and well even with mobile devices everywhere.
We rarely see complete displacement. We see convergence and coexistence.

Agentic AI challenges the SaaS layer, especially when it comes to repetitive UI-based tasks.
But that does not mean SaaS is going away. The smart vendors will adapt by going deeper, offering more specialization, and building experiences that AI agents cannot easily replicate.
At the same time, agentic AI platforms will need to evolve.
They must be trustworthy, adaptable, and capable of handling the complexity of real business logic.
Conclusion
The companies that move fast will not choose between SaaS and agentic AI.

They will use both, combining the power of autonomous agents with the structure and trust of well-designed software.
Agentic AI is not the end of SaaS.
It is the start of a smarter, more adaptive version of it.